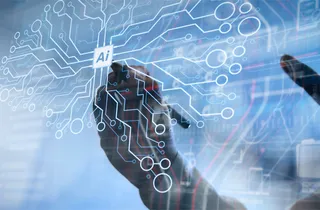
Overcoming bias in artificial intelligence
The world looks different now compared to early 2020. The pandemic has heightened organisations’ reliance on technology to complete tasks, as well as the challenges of attracting and retaining staff. It has called for new ways of working that are likely to become permanent for many.
Artificial intelligence (AI) is among the technologies playing a growing role in helping organisations operate. It can collect insights about a business, track customer patterns and make recommendations that, ideally, lead to better-informed actions.
Even before the COVID-19 pandemic, AI was being applied to a range of industries in an effort to reduce errors and lessen the need for human labour to complete tasks ranging from driving vehicles to performing surgery. It was also supporting less serious decision-making—helping marketers track the success of their campaigns, for example, or alerting retailers to potential fraud or problems with the customer journey.
Now, organisations are seeking out such AI-driven benefits not only to thrive but to survive. According to new research by Capital Economics that was commissioned by the UK government, business adoption of AI in the UK is expected to grow from 15.1 percent in 2020 to 22.7 percent in 2025. This equates to an additional 267,000 businesses adopting AI in their operations during that time. By 2040, the research predicts overall adoption of AI to reach 34.8 percent, with 1.3 million UK businesses using the technology.
“The pandemic has changed the competitive landscape for many businesses and given them new challenges to manage, ranging from supply shortages to employees with new expectations about work,” said Toby Harris, technology practice leader at Travelers Europe. “AI applications have the potential to ease these burdens and offer a competitive advantage—but they also generate new risks that must be assessed and managed.”
“The human element may introduce bias in AI models, but it is possible to identify and limit that bias.” Toby Harris, Travelers Europe
Better data-driven decision-making
One of the risks of AI applications is bias. If the unconscious biases of the people developing and training AI models aren’t managed, the models will make skewed decisions—perpetuating existing human biases and potentially creating new ones. That could inadvertently restrict the demographics of people an organisation recruits and hires, or influence which customers are given more favourable contract terms.
In recent years, such biases have surfaced in a wide range of industries—in AI algorithms used by Amazon that showed a preference for male candidates for employment, for example, and in software widely used in the US healthcare system that called for black patients to receive a lower standard of care. As a Venture Beat report indicates, faulty AI can cause serious reputational damage and costly failures for businesses that make decisions based on erroneous, AI-supplied conclusions.
On the surface, it might seem that AI should help weed out human biases and not perpetuate them. After all, since machine learning algorithms learn to consider only the variables that improve their predictive accuracy based on the training data used, they may also reduce humans’ subjective interpretation of data. As the MIT research scientist Andrew McAfee has said, “If you want the bias out, get the algorithms in.”
Sometimes less-biased decisions do result. A study referenced in a McKinsey report found that automated financial underwriting systems particularly benefit historically underserved applicants.
But an AI application is only as good as the quality of the data feeding it and the quality of the model used to train it to make decisions—and a growing body of research reveals existing flaws. The technology research and consulting firm Gartner predicts that through 2022, 85 percent of AI projects will deliver erroneous outcomes due to bias in data, algorithms or the teams responsible for managing them.
The good news is that we know the significance of the problem—and as awareness grows with regard to AI’s flaws, new auditing systems will be designed to better manage bias and limit the impact of that bias on decision-making. Better-informed actions can follow, since unlike human decisions, the recommendations suggested by AI can be objectively examined and interrogated.
To manage the risks of AI bias, insurers will be monitoring AI applications and calling for customers to have controls in place that pave the way for continuous improvement. Organisations that currently use AI models or are considering adopting them in the coming years can ask themselves these questions, suggested in a report by Appen, to help:
1. What business challenge are we trying to solve?
Be as narrow and specific as possible to ensure your model is addressing the precise problem you’re wanting to solve.
2. How good is our algorithmic hygiene?
Better-quality inputs generate better-quality outputs. So before using an AI model, ensure you have a strong understanding of the various kinds and causes of bias, as well as of your intended audience.
3. Does a broad enough range of perspectives inform our data collection process and our model?
Have people with a diversity of opinions and perspectives dissect each data point and interact with the model. Their questions and comments will be (and should be) different—and they can help you enhance your model’s flexibility and avert problems down the line.
4. Is there bias hidden in this data?
Your data may have classes and labels that introduce bias into your algorithms. You can minimise the potential for bias by scanning for objectionable labels and ensuring your data accurately reflects not your team, but the diversity of your end users.
5. Are we including diverse viewpoints to help retrain the model on an ongoing basis?
A model can become more biased over time if it is not retrained with input from a diverse, multidisciplinary team.
6. Have we given end users the opportunity to provide feedback?
User comments in the testing and deployment phases will help ensure your model is performing well and providing assistance to solve real-world problems.
7. Are we collecting the right mix of feedback to continuously improve our model?
Consider input from customers and other end users, as well as independent auditors who could identify biases you may have missed or offer suggestions to improve your model’s accuracy and overall performance.
Sources: Appen, 7 June 2021; AI Multiple, 22 January 2022
“The human element may introduce bias in AI models, but it is possible to identify and limit that bias,” said Harris. “From an underwriting perspective, insurers will look for organisations to take a thoughtful approach to managing AI bias and the risks it can pose.
“That should involve making a thorough assessment of the business need you’re addressing and for whom, identifying a team with a diversity of perspectives who can analyse the data used to train the model and the recommendations it suggests, and developing a plan to continuously review and improve the model based on that information.”
Already registered?
Login to your account
If you don't have a login or your access has expired, you will need to purchase a subscription to gain access to this article, including all our online content.
For more information on individual annual subscriptions for full paid access and corporate subscription options please contact us.
To request a FREE 2-week trial subscription, please signup.
NOTE - this can take up to 48hrs to be approved.
For multi-user price options, or to check if your company has an existing subscription that we can add you to for FREE, please email Elliot Field at efield@newtonmedia.co.uk or Adrian Tapping at atapping@newtonmedia.co.uk
Editor's picks
Editor's picks
More articles
Copyright © intelligentinsurer.com 2024 | Headless Content Management with Blaze