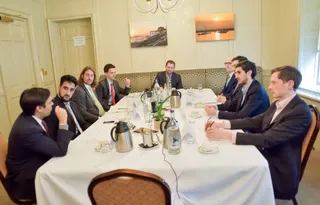
Artificial intelligence: a game-changer for risk transfer
In attendance:
- Irfan Akhtar, head of ReMetrica for Life, Health & Pensions, Aon Analytics
- Greg Brown, founding partner, Oxbow Partners
- Ben Canagaretna, group chief actuary, Barbican Insurance Group
- Craig Civil, head of data innovation, Lloyd’s
- Adrien Cohen, co-founder, Tractable
- Paul Delbridge, London Market insurance leader, PwC UK
- Adrian Rands, CEO, QuanTemplate
- Will Thorne, innovation leader, The Channel Syndicate
- Chair: Wyn Jenkins, Intelligent Insurer
The pressure on companies to explore AI—is this internal or external? Have large budgets been allocated?
Will Thorne: It’s very difficult to speak on behalf of other insurers and reinsurers, so I won’t try! We set up the innovation division at Channel about a year and a half ago, and various other innovation initiatives have been introduced across the SCOR group since then. There is a tremendous awareness at board level and throughout the company of how technology can change what we do, and exploring artificial intelligence (AI) is a part of that.
There are a lot of different use cases for AI which all come with their own challenges. It’s easy to engage in a proof of concept with someone like Tractable around a specific use case using an external AI model, but it is more difficult for insurers to embed AI within our normal day-to-day processes.
Ben Canagaretna: Our company is quite small in contrast to SCOR, but we like to think of ourselves as entrepreneurial and innovative. We’ve only really scratched the surface as an industry. A lot of it is inertia driven by protectionism, because it’s not in people’s interests to change and for a long time the industry has been very profitable.
What we’re seeing now is a softening market. Margins are getting squeezed and people are looking harder at their bottom line and how they can generate margin.
These techniques will become a lot more prevalent as people look at new ways of trying to drive bottom line profit that are different from how they’ve done things in the past. We’re investing a lot of time—maybe not a lot of money at this stage—but as we start to get results, I see a growing momentum and a growing budget within Barbican to put us in this area.
Craig Civil: There is definite recognition that AI and machine learning has a role to play. One of my jobs is to make that a practical reality and identify opportunities for Lloyd’s Corporation to use technology and AI to make us more efficient and also to provide some leadership and support to the market to enable them to identify opportunities.
We’re engaging with partner organisations, so we’re not going to do this by ourselves. We’ve started to build small scale from both budget and time perspectives, with a proof of concept using AI. We’ve defined success measures at the end of that so we know whether we’ve succeeded or failed and the path that we then need to take.
Adrien Cohen: We’ve been mostly present in the US last year and now we’re starting to engage with insurance companies in the UK. It moved much faster in the US, but the companies we were working with were allocating budgets and resources trying to make it work.
We need the ability to insert those products in the workflow and capture the value that is there in theory. This is something we’ve been able to push in the US and now here in the UK. We are seeing more demand, but not just trying the proof of concept of how AI could be. An increasing number of insurance companies want to deploy AI in their processes and structure a team around that and have proper budget allocated to trialling AI.
It’s still very early stage, but we’re seeing the UK moving quite fast in this space.
Is the US ahead of the UK?
Cohen: The UK is catching up. In the US we’re mostly present in the motor insurance segment; it’s a profitable business so there’s probably more flexibility into looking into new technologies. In the UK there’s a lot of pressure on this segment and in Europe in general it’s simply much more competitive, so insurance companies need to look into ways to start generating savings. At the same time they have less financial budget because of the pressure on prices and the bottom line.
Irfan Akhtar: The bigger players who are profitable and happy where they are at the top of the food chain are waiting for something to give them that pressure, whether it’s competition or another equally sized payout. We see it with the smaller, more flexible players or startups trying to steal some business but until they get to that critical mass position, the bigger players might not move.
From people we’ve been speaking to, the board level had given them a mandate to at least find out what’s going on so we’re not left high and dry if somebody discovers something that will be a major shift allowing them to steal business away.
There is a knowledge gap between traditional actuaries and what AI entails. It’s big data, the internet of things (IoT) and AI combined, and knowing how to capitalise on it. Direct Line, for example, has a data scientist rather than an actuary at the head of that part of the business.
What is the broker’s point of view? Is it something to embrace because the role is changing?
Akhtar: A bit of both. We offer to look at it for clients who don’t know where to start, with a team who look at the data and see if they can improve their current reserving, pricing and marketing factors. On top of the usual rating factors, we see what other data you have that can make groups more homogenous and price in a better way.
At the same time, we’ve got tonnes of data. AI can now automate many tasks we previously thought were the domain of humans because it involves “thinking”. We’re looking at those areas across the business to make savings.
Thorne: I find it very interesting that you say one of the roles of using AI rating is to make groups more homogenous, because the classic outcome of AI is that you end up with a heat map with hotspots of correlation around very small groups, enabling price matching at a more granular level.
There’s a much wider discussion in the industry about whether you end up with a product designed for an individual rather than a group, and what the consequences of that are.
Greg Brown: I’m interested in the ‘fear versus reality’ of the insurance industry’s response to AI. In the last 12 months most board level people we’re talking to are saying ‘AI is going to eat my lunch, help’. A lot of what is driving this is the external influence of insurtech. There is a fear of the insurtech ‘noise’ in the background.
To what extent are the questions being asked around trying to solve real business problems, versus a fear of the unknown and wanting to respond to it?
Adrian Rands: What we see is that most organisations, operation wise, are converting human tasks and cutting through bottlenecks in operational processes and using pretty straightforward machine learning techniques that have recognised what humans have done elsewhere in the organisation and implemented that.
In the life sector, we’ve got clients who have been pretty successful in that area. The rollout of AI is primarily looking at enhancing the efficiency of what currently operates and the opportunity to capture more business or to take business from competitors, but it’s still in the innovation lab stage.
Canagaretna: It’s not necessarily AI that is scaring people, it’s technology more generally. The role of the broker and the underwriter is becoming more blurred and the way business is accessed via distribution channels is becoming more direct, and that means individuals are being cut out of the chain. What people are uncertain about is how that evolution will manifest itself over the next 10 years and what is their role, if any, in the supply chain?
What about the other technology buzzwords?
Brown: AI often gets pegged on to something else such as blockchain.
Thorne: Everyone wants to use the buzzwords but what we’re really trying to understand as an insurer is how business is going to look 10, 20 years from now, and how we can equip ourselves to understand and adapt.
The job of people like me is to say ‘this is what’s happening, here’s the result of engaging with this particular piece of new technology, and this is why we think it can add tangible value to the business’. That enables boards to make strategic decisions.
Cohen: We need to be more specific in terms of the use cases we’re talking about. For instance with motor, we’ve trained AI to look at photos of car damage and make an assessment of the damage, which is a step that is adding a lot of friction today in the claims process. You can think of the same thing in property.
If you’re a desktop engineer, the first reaction you’re going to have is that it’s scary, but if you’re the claims director or the C-level, you’re thinking about efficiency and making sure you have consistency in your claim appraisal process. The way we see this technology bringing value, rather than automating tasks, is that it is augmenting humans to do the job faster and in a smarter way, to be more focused on meaningful and complex tasks.
There are several processes you can automate, but you can’t automate the entire value chain. A lot of education is required around who is using this technology and how it is supporting and augmenting your team rather than replacing them.
Civil: That supports how we’ve approached it. Naturally, there was a fear in terms of why you’re engaging with this supplier or do you just want to get rid of jobs. But when you engage the people who are potentially impacted, and they start to see the results coming through they get really excited. They realise that a machine can take away the drudgery, and they can focus on the complex questions from the market that make their job more interesting and fulfilling.
Brown: There has to be an efficiency gained somewhere otherwise you wouldn’t do it. Either to allow you to do more with the same, which would mean no job losses, or to take cost out which would have job implications. They must have a belief that it will allow growth.
Paul Delbridge: If you say that you’re taking away the drudgery, and what we want to do is liberate underwriters to grow the business, train people, and grow the top line, improve margins and new opportunities, that’s a very powerful way of selling AI. Otherwise, it’s a case of ‘you are eliminating my job’.
Thorne: You have to differentiate between light-touch underwriting in retail products as opposed to commercial and specialty lines, where underwriting a risk is actually quite a long process. In those lines the opportunity from technology is all about enhancing how underwriters look at a risk. SCOR’s tagline is ‘the art and science of risk’ and the more we can empower our underwriters to use technology and data, the better.
Civil: For us it’s about making sure we’re offering a consistent high quality service. If you give the machine the same inputs you’re going to get the same answer out of it, whereas with a mixed team you can sometimes get inconsistent results. The more you get machines involved in the decision-making processes, the more you can offer a guarantee for quality of services.
Brown: That’s the difference between AI and automation. Automation is ‘give me the same inputs and I’ll give you exactly the same outputs’, whereas AI, over time, is ‘give me the same inputs and I will have learned and I will give you different outputs’.
We’re debating whether it continually learns in an uncontrolled way or whether there is governance around it. You might have monthly review cycles where you ask if you are happy that it will make these new decisions.
Thorne: One of things we debated on the FCA Insurtech Panel is you have to be able to explain to a consumer why they’ve been rejected for insurance if a ‘black box’ AI is making those decisions. You have to empower people to understand what the model is actually doing.
Civil: Yes, and understand what the software is doing. Lifting the bonnet on what’s going on underneath so you know and can trace how it’s come up with the answer and then teach it to get it right the next time.
Delbridge: In traditional generalised modelling roles, it’s quite specific. But with machine learning, you can have a number of non-linear models which you have blended, which makes it quite difficult for an insurer to explain why your premium is higher.
Akhtar: That’s going to be the reason for hesitation for traditional people embarking on this type of process, because of the unknown. On the governance side, other jobs can be created with this new system in place but there is a very real risk of job losses. We’ve seen Fukoku Life Mutual replacing 30 jobs with an AI system, and those things catch headlines so fearmongering is going to come out.
I don’t think companies know the full cost and efficiency savings that can come from AI, but they certainly have an idea it can help so will pursue it. A lot of the tasks can be automated, and they will impact underwriting—whether it’s initial or claims underwriting.
Canagaretna: My view is that the role of the insurer will evolve with much more emphasis on value-added service such as active risk mitigation rather than just giving an insurance contract and charging a premium. We’ll be more engaged with our customers around how they set their businesses up in a way to minimise risk, so that they pay a more efficient premium.
The data analytics side of the business is also going to grow. I don’t believe we’re going to be businesses with one big red button and everyone presses it in the morning and then comes back in the afternoon to see what the outcome is. There’s always going to be the need for intelligent interpretation of the output and communication of that to investors, policyholders and regulators.
If we get our arms around it we can push the London Market forward to the front ofthe queue, and bring in more premium volume.
Do stories like Fukoku hold back the industry? You pitch it to the boards in terms of savings and growth, but is there reservation from external perceptions?
Brown: There’s holding back because of an internal fear of job losses, changing the industry, and value chain compressions that you were talking about. But then there’s PR fear which to me seems distinctly separate.
Delbridge: The people who recognise the writing on the wall and embrace AI will get the opportunities to retrain. It’s a very fair point talking about the fear or the kind of PR profile that you can have. But humans do make mistakes and if AI can be positioned in such a way that it will make very consistent decisions when handling a claim: ‘we’ll do it without error and get it right the first time’, people will be less fearful of that.
Cohen: There are simple cases or claims that you could settle in minutes, and then there are more complex cases that would be pushed to experts.
Some jobs will disappear as with any new technology, some jobs will be created and it’s hard to predict what kind of jobs we will need. How to manage and oversee this transition, and how to make sure that the benefits of automation are shared among everyone is important.
Akhtar: I don’t think jobs are going to go completely, but many jobs are doing the drudgery. The net amount of jobs might go down but there might be more jobs created that we can’t even imagine right now.
The reality is that for AI to be used, it has to be at least performing at the human level or better.
Brown: If you’re looking at cost efficiency, it just needs to be efficient at certain elements. The bit that is automated has to be better than the human performing the same task.
Cohen: AI can add a lot of value and change your claims process because it’s instant. Maybe it’s less accurate than a human, but because it can provide a decision instantly—just looking at photos—you can come up with a new service to the policyholder and the overall value-add is bigger even if it’s not yet at human performance.
You have a scale component as well: AI can process thousands of claims or thousands of photos, or whatever data is being used, 24 hours a day. When you do have the accuracy bit—in particular for medical imagery—the consistency and the accuracy of the decision-making is more important than the speed and scale.
When we look at use cases of how to bring technology to market, we always assess whether there is value added in speed, scale or accuracy.
Rands: Insurance practitioners, particularly in the London Market, are highly skilled at what they do so their motivation is to ensure that the extent of their knowledge is incorporated in mechanical process and ultimately, machines aren’t as sophisticated as the human brain.
The key for AI being implemented successfully is to ensure there’s that control aspect for the management of the system and that they have the user experience and the user ability to be able to influence the algorithm. Ultimately insurance companies and practitioners don’t trust the black box. What they want is a greater set of tools to be able to perform their jobs more efficiently and effectively.
Brown: AI at the moment typically does one thing very well, but what humans are very good at is seeing the bigger picture and a detailed action within a bigger context. The main reason AI still requires human intervention, to the point about governance, is that it doesn’t foresee all the possible unintended consequences of the one narrow focus that it has.
Once you have replicated a whole human and its intelligence, then in theory that doesn’t need any human governance. But I think we’re far away from that.
Rands: If you look at where AI is being implemented for an insurance work flow, it’s in areas of data quality or claims processing. The existing framework already has a series of logical steps that if the content is simple, it goes down a streamlined process and if it’s complicated it then gets put into a more bespoke set of analysis which is human. Where AI sits in that is essentially extending the quantity of business that can go down the automated route.
Civil: I worked with the IBM Watson health team to create one of the Watson products, and nurses and doctors started off not liking the idea of working with a machine. Gradually, they realised it wasn’t challenging them but rather helping them.
It can take away some of the routine questions, releasing the staff to ask more specific questions that wouldn’t have come to the fore unless there was a machine churning through the basics.
Cohen: Medical imagery is one of the places where we need this kind of technology. If you need a scan you’d rather show up at 9am than 6pm, because at 6pm the radiologists have spent hours looking through hundreds of scans—they are tired and make mistakes.
Having the support of AI to ensure that the diagnostic evaluation is consistent—whether you show up at 9am or 5pm—will bring a lot of benefits.
A mammogram is one of the easiest because it’s just two scans, so you can learn this task pretty fast, whereas a MRI scan is more complex. In the medical space you have much more regulation so it has to be supporting the doctors.
Civil: Going back to the London Market, one of the interesting things I found is that the healthcare aspect worked because of data quality—the data being captured was granular and of good quality. We need to do similar work in terms of improving the quantity, the quality and the governance around the data we’re collecting.
Delbridge: One of the things you hear is that we have a real problem matching policy data with claims data. If you could digitise all the paper records and allow AI to sift through them, this is a job that would take humans decades to do.
The more positive stories you hear of AI doing a job that simply can’t be done in a sensible amount of time by humans, the more you will start to see people recognising the benefit.
Akhtar: Another use case is dealing with reserving requirements. For people with disabilities who have regular medical check-ups, insurers usually just reserve until the end of the contract, so they’re holding a massive reserve for these people who might be on their way to recovery, based on the doctor’s report.
An AI could churn through and give a correct reserving factor for these patients, whereas human manpower will not cover it. Just like the digitisation of paper records, AI is generating massive economic value around the world based on supervised learning, which needs tonnes of data to help it learn. Google and Amazon have a lot of that data. A single insurance company may not have enough to train the system up, so what incentive will there be to share data across parties to build this AI?
Where do you see the technology being applied to sectors of the insurance industry?
Canagaretna: The personal lines industry is ahead of commercial lines—machine learning techniques for pricing are being used fairly widely
Generalised linear models are being replaced by machine learning techniques over time. The big challenge at Lloyd’s and for commercial lines is getting the right data in the right form so you can start applying these techniques.
Supplementing that data with more external data sources will open this up even further.
Thorne: Many of the AI use cases under discussion at the moment are focused on bringing in external data. You can start doing things around understanding event frequency better than just using internal data and models, and that’s one way of getting people excited about what AI can do.
You can say ‘you’ve been pricing for the company based on four or five key data points for the last 20 years; adding these two which are available by scraping public data and running a model through them can transform how you do business’.
Delbridge: Sensor technology provides a wealth of stuff you otherwise wouldn’t have had. Five years from now I wouldn’t be surprised if insurers are starting to take direct feeds from their insureds and able to give genuine credit for advances in risk management policies which currently are a bit more difficult to quantify.
Thorne: I’m always surprised when I hear consultants pushing this idea because if you talk to large corporates, they are often unwilling to share that level of granular data. Real-time data feeds would require their approach to change.
Rands: It’s also a challenge well beyond where the industry is now. Fortunately for the cornerstone of the London Market Target Operating Model, structured data capture and the Placing Platform Limited are all about creating consistent, confirmed, reliable and available data. Enriching and augmenting it is the cornerstone of what we do at QuanTemplate.
Cohen: Access to data is definitely one of the biggest limitations. It’s great to hear people from the industry thinking about pooling their data.
We have decided to build a partnership with one player who has a huge amount of data, consolidating all the photographs of cars coming from body shops, so we don’t need insurance companies’ data for our product to work. They feel very happy about it because they’re getting a commercial preference in return. They are great partners.
Long-term there is a question of pooling everyone’s data to build an even better AI, but for now we make sure that data is separate from one insurance company to another and that they have control on where the data sits.
Canagaretna: At Barbican, we’re are big on corporate partnerships. A lot of our capital comes from third parties all around the world, but increasingly we’re starting to look for corporate partnerships with technology companies because we see that as the future. We may not have big budgets at our disposal, but we can add a lot of value on the intellectual side and bring our insurance expertise to the fold.
Thorne: It’s definitely a trend. When we set up the innovation department for Channel, we were one of the few companies in the London Market who had a dedicated function for innovation. Now I think there’s more than 10. XL Catlin is even hiring a dedicated AI specialist.
What’s changed in the last two years? Was there a big breakthrough?
Rands: Recognition of where it can add value today on the cost side. The application of AI for optimising processes is a no-brainer, especially if you look at the return on investment.
Cohen: It’s definitely the breakthroughs that have happened in the last two years that pushed us to build this company. It was only in 2015 that computers surpassed human accuracy on image recognition tasks.
Brown: Going back to mammography, is it true that in certain circumstances it was better than humans?
Cohen: No, because it was not deep learning. Only deep learning for computer vision gives us the ability to cope with variation in terms of background, angle, lighting and to analyse images.
For the first time there is one task where AI is better than humans, which was driven by deep learning and also by improvement in terms of computing power. We’re focusing on computer vision because it’s the one task that works better than with a human, provided you have enough data.
Canagaretna: There’s a lot more money flowing into insurtech over the last few years and that’s due to a number of factors outside the insurance industry. People are looking to deploy investments and are struggling to find acceptable returns. Insurtech has been identified as a huge opportunity to transform.
Akhtar: Another thing is the way that the algorithms work; the explosion of data in recent years made everything possible. Some of the techniques were around before, but without the data we couldn’t get it to the threshold required for good performance.
Civil: There’s an element of catch-up in the London insurance market and we hope we’re going to benefit from the work that’s been done before.
Delbridge: Regulatory compliance has become more of a headache and there’s a lot of ‘regtech’, but a lot of the time these present the regulatory rules and basically sift through your intranet and email system and compare the rules and internal policies side by side to identify non-compliance. With the right monitoring—probably leveraging AI—we could have avoided the Libor fixing scandal.
Thorne: For driverless cars, when you ask whether new products can be created, the question is whether insurers will be willing to insure software breakdown, particularly if it’s the neural network powering the decision-making embedded in driverless cars or something similar.
Akhtar: One of the interesting aspects is insuring the previously uninsurable. Areas that normally wouldn’t be insurable become insurable because of sharing data, real-time interaction and analysing data.
And cyber?
Akhtar: When you digitise everything there are more opportunities for cybercrime but Stroz Friedberg, an Aon company, mentioned that 90 percent of cyber breaches are due to human weakness rather than the computer system’s weaknesses. It’s the human element that attackers exploit.
Brown: If you’re talking about a lot more data and IoT, the data is outside and in the public domain, whether encrypted or not. It creates a lot more opportunity for people to get hold of the data that they shouldn’t have and therefore it creates risk.
Canagaretna: As we become more sophisticated about managing our risk in the more developed countries, insurance penetrations will naturally become higher and cyber will obviously be a key growth area.
Can AI roll out simpler underwriting technique?
Canagaretna: Technology more generally is an enabler rather than AI specifically. Broker portals have become more prevalent, so the ability to automatically quote, price and give your document to a customer in seconds rather than going through the cumbersome process that we have in certain classes, opens up the possibility of distributing more business to certain countries.
Delbridge: Particularly microinsurance in Africa: you couldn’t do that cost-effectively five to 10 years ago but now, why not?
Thorne: One of my frustrations with these discussions is that we often end up talking about how AI is going to solve the protection gap, how it’s going to transform the way we do business. Today we have actually spent most of our time talking about a series of very discrete use cases.
Our approach is to be very practical about what we think a specific piece of technology applied to a specific use case can do. Otherwise you get to the situation where ‘blockchain will solve marine hull insurance’.
Akhtar: For self-driving cars, insurance companies need to consider what to charge. Tesla recently mentioned that he’s not getting the right rates from the insurance industry, as actuaries want to wait and see what the risk is.
The tests might show it’s safer or better than human driving, but what is the actual risk? What rating factors and premiums should be appropriate for that? Normally, we wait for the experience to pan out to be able to price it, but in this case, Tesla said that because they don’t think they’re going to get the right rates, they might self-insure.
This gives opportunities to startups and insurance companies if the big players don’t want to go down that route. We have to consider how do we change or adjust the premium for something where there’s an AI element, and we might not have the luxury of waiting for the experience to pan out long enough as other players may jump at the chance.
Thorne: Another issue for re/insurers is potential asymmetries of information, for example if corporates decide not to share certain information regarding their own adoption of AI. If there is a fundamental change in an industry which we are insuring or reinsuring, we need to truly understand how our clients are managing their risk.
Already registered?
Login to your account
If you don't have a login or your access has expired, you will need to purchase a subscription to gain access to this article, including all our online content.
For more information on individual annual subscriptions for full paid access and corporate subscription options please contact us.
To request a FREE 2-week trial subscription, please signup.
NOTE - this can take up to 48hrs to be approved.
For multi-user price options, or to check if your company has an existing subscription that we can add you to for FREE, please email Elliot Field at efield@newtonmedia.co.uk or Adrian Tapping at atapping@newtonmedia.co.uk
Editor's picks
Editor's picks
More articles
Copyright © intelligentinsurer.com 2024 | Headless Content Management with Blaze